Learn which stocks the world's top hedge fund managers are looking at investing in right now
Join 1000+ other investors who get access to behind the scenes investing strategies & shareholder letters from leading Hedge Funds
What Is a Correlation Coefficient?
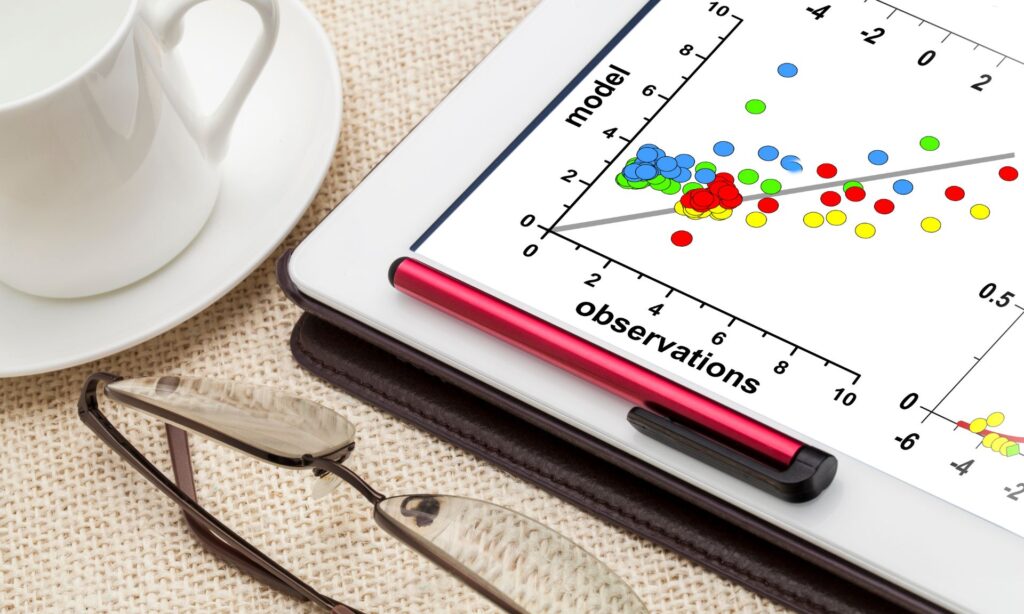
A correlation coefficient is a statistical measure that quantifies the strength and direction of the linear relationship between two variables. It is a value that ranges from -1 to 1, with -1 indicating a perfect negative correlation, 0 indicating no linear relationship, and 1 indicating a perfect positive correlation.
The most common type of correlation coefficient is the Pearson product-moment correlation coefficient, also known as Pearson’s r. This coefficient measures the linear association between two variables, x and y, and is calculated by dividing the covariance of the two variables by the product of their standard deviations.
The formula for Pearson’s correlation coefficient is:
r = (Σ(x – x̄)(y – ȳ)) / (√(Σ(x – x̄)²) × √(Σ(y – ȳ)²))
Where:
- r is the correlation coefficient
- x and y are the individual data points for the two variables
- x̄ and ȳ are the means of the two variables
- Σ represents the sum of the terms in the numerator and denominator
READ ALSO: Compulsory Convertible Debentures (CCDs): A Unique Financing Tool for Businesses
Understanding Correlation Coefficients
Correlation coefficients provide valuable insights into the relationship between two variables. Here’s a closer look at the different types of correlation and what they mean:
- Positive Correlation (r > 0):
- Indicates that as one variable increases, the other variable tends to increase as well.
- The closer the coefficient is to 1, the stronger the positive correlation.
- For example, a positive correlation of 0.8 between stock price and company revenue suggests that as a company’s revenue increases, its stock price tends to rise as well.
- Negative Correlation (r < 0):
- Indicates that as one variable increases, the other variable tends to decrease.
- The closer the coefficient is to -1, the stronger the negative correlation.
- For instance, a negative correlation of -0.7 between temperature and heating bills suggests that as temperatures rise, heating bills tend to decrease.
- No Correlation (r = 0):
- Indicates that there is no linear relationship between the two variables.
- This means that the variables are independent, and changes in one variable do not systematically relate to changes in the other.
- For example, a correlation coefficient of 0 between the number of sunspots and the price of gold suggests that there is no linear relationship between these two variables.
It’s important to note that a high correlation coefficient (close to 1 or -1) does not necessarily imply causation. It simply means that the two variables are linearly related, but it does not determine which variable is the cause and which is the effect. Additional analysis is required to establish a causal relationship between the variables.
Using Correlation Coefficients in Finance and Investing
Correlation coefficients are widely used in finance and investing to analyze the relationships between different financial assets, such as stocks, bonds, and commodities. Here are some common applications:
- Portfolio Diversification:
- Investors often use correlation coefficients to assess the diversification potential of their portfolios.
- Assets with low or negative correlation can help reduce the overall risk of a portfolio, as their price movements tend to be independent or inversely related.
- Factor Investing:
- Correlation analysis is a key component of factor investing, which involves constructing portfolios based on specific factors (e.g., value, momentum, size) that are associated with excess returns.
- Investors analyze the correlation between these factors and various asset classes to identify potential investment opportunities.
- Quantitative Trading:
- Quantitative traders use historical correlation data to anticipate and capitalize on changes in asset prices.
- By understanding the relationships between different assets, they can develop trading strategies that exploit these correlations.
- Risk Management:
- Correlation coefficients are used in risk management to measure and monitor the overall risk of a portfolio.
- Portfolio managers may adjust their holdings based on changes in asset correlations to maintain an appropriate level of diversification and risk.
READ ALSO: Debentures: A Comprehensive Guide to Understanding the Debt Instrument
Learn which stocks the world's top hedge fund managers are looking at investing in right now
Join 1000+ other investors who get access to behind the scenes investing strategies & shareholder letters from leading Hedge Funds
Calculating Correlation Coefficients in Excel
Calculating correlation coefficients can be a time-consuming task, especially when dealing with large datasets. Fortunately, many software applications, such as Microsoft Excel, provide built-in functions to simplify the process.
In Excel, you can use the CORREL() function to calculate the Pearson correlation coefficient between two data sets. The syntax is as follows:
=CORREL(array1, array2)
Where:
- array1 and array2 are the two data ranges you want to analyze.
Alternatively, you can use the Data Analysis ToolPak in Excel to generate a correlation matrix for multiple variables. Here’s how:
- Go to the Data tab and click on Data Analysis.
- Select Correlation from the list of available analysis tools and click OK.
- Select the range of data you want to analyze and check the “Labels in first row” box if your data has column headers.
- Choose the output location for the correlation matrix and click OK.
The resulting correlation matrix will display the pairwise correlation coefficients between all the variables in your dataset.
Conclusion
Correlation coefficients are a powerful tool for understanding the relationships between variables in a wide range of fields, including finance, economics, and scientific research. By quantifying the strength and direction of these relationships, correlation coefficients provide valuable insights that can inform decision-making, risk management, and investment strategies.
Whether you’re an investor, a researcher, or simply someone interested in data analysis, understanding the fundamentals of correlation coefficients and how to interpret them is an essential skill. By mastering this concept, you can gain a deeper understanding of the world around you and make more informed choices based on the information at hand.
FAQs about Correlation Coefficients
What is the difference between correlation and causation?
Correlation measures the strength and direction of the linear relationship between two variables, but it does not imply causation.
Causation refers to a cause-and-effect relationship, where changes in one variable directly cause changes in another variable.
Just because two variables are correlated, it does not necessarily mean that one variable causes the other. Additional analysis is required to establish a causal relationship.
What is considered a strong correlation?
The interpretation of correlation strength varies depending on the field of study and the specific application.
In general, a correlation coefficient above 0.7 (positive or negative) is considered a strong correlation, while a coefficient below 0.3 is considered a weak correlation.
In the social sciences, a correlation coefficient of 0.5 or higher is often considered a strong relationship.
Can correlation coefficients be used to make predictions?
Correlation coefficients can be used to make predictions, but they have limitations.
Correlation coefficients only measure the linear relationship between two variables and do not provide information about the underlying nature of the relationship.
To make accurate predictions, you would need to use regression analysis, which models the relationship between the variables and allows for the estimation of future values.
How do you interpret a negative correlation coefficient?
A negative correlation coefficient indicates an inverse relationship between the two variables.
As one variable increases, the other variable tends to decrease, and vice versa.
The closer the coefficient is to -1, the stronger the negative correlation, meaning the variables move in opposite directions with greater consistency.
Can correlation coefficients be used to compare the strength of relationships across different datasets?
Yes, correlation coefficients can be used to compare the strength of relationships across different datasets, but with some caveats.
The datasets should have the same scale and units of measurement, as correlation coefficients are sensitive to the scale of the variables.
Additionally, the sample sizes should be comparable, as larger sample sizes can lead to higher correlation coefficients, even if the underlying relationship is the same.
In another related article, What is a Family Trust and How to Set One Up
Want to see what stocks the top hedge funds are looking at BEFORE it hits the mainstream news? Get free access for 7 days