Learn which stocks the world's top hedge fund managers are looking at investing in right now
Join 1000+ other investors who get access to behind the scenes investing strategies & shareholder letters from leading Hedge Funds
The world of stock trading and investing has been fundamentally disrupted by the emergence of artificial intelligence (AI) technologies. AI-powered tools and algorithms are automating processes, enhancing analysis capabilities, and driving new levels of speed and efficiency in financial markets.
In particular, the field of algorithmic trading has experienced explosive growth thanks to AI advancements. Algorithmic trading refers to using computer programs to automate and execute trading strategies in financial markets. It enables trades to be executed much faster and more frequently than human traders could manage manually.
This comprehensive guide explores the transformative impact of AI on stock trading and the ascent of algorithmic investing.
The Evolution of Algorithmic Trading
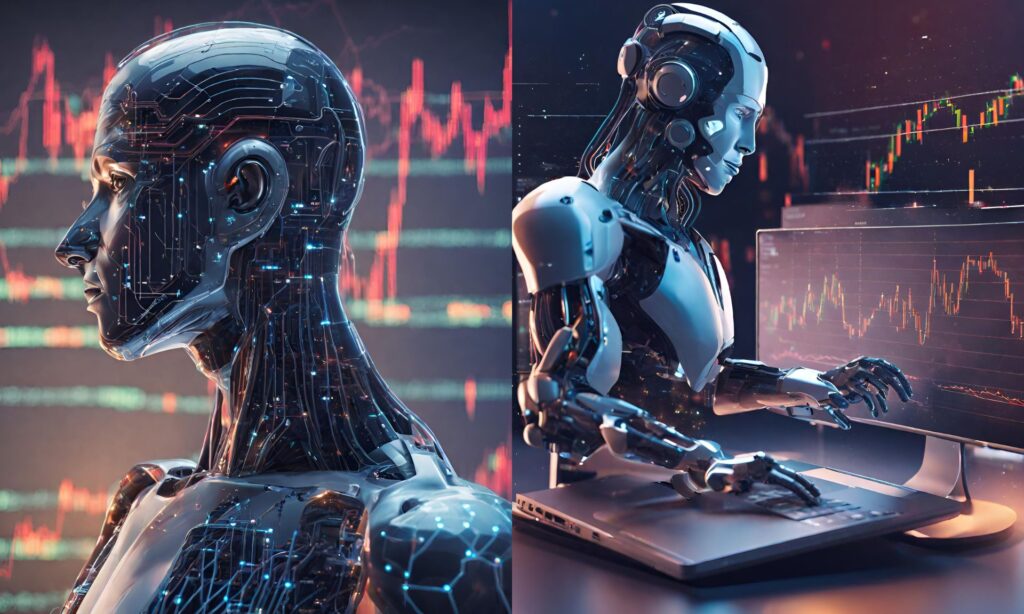
Algorithmic trading has its origins in the 1970s, when early computerized trading systems began emerging. However, it only gained mainstream traction in the 1990s and 2000s as online trading platforms became prevalent.
In traditional stock trading, human traders manually monitor markets, analyze data, and decide when to buy or sell securities. Algorithmic trading automates this process by using computer programs to follow predefined trading rules and models.
Key milestones in the evolution of algorithmic trading:
- 1970s – Early computerized trading systems emerge.
- 1980s – Development of trading strategies based on mathematical models.
- 1990s – Online trading platforms enable algorithmic trading to flourish.
- 2000s – High frequency trading driven by algorithms becomes widespread.
- 2010s – Machine learning algorithms transform algorithmic trading.
Today, over 80% of all stock trading volume in the US is driven by algorithms rather than humans directly placing trades.
How AI is Revolutionizing Algorithmic Trading
Artificial intelligence has taken algorithmic trading to unprecedented levels in terms of speed, predictive accuracy, and complexity.
AI capabilities like machine learning and neural networks have enabled a new generation of “smart” algorithms that can analyze vast amounts of data, continuously improve over time, adapt to changing market dynamics, and outperform predetermined algorithms.
Key ways AI is transforming algorithmic trading:
Learn which stocks the world's top hedge fund managers are looking at investing in right now
Join 1000+ other investors who get access to behind the scenes investing strategies & shareholder letters from leading Hedge Funds
Advanced Data Analysis
- AI can process infinite combinations of market data at astonishing speeds to uncover hidden insights.
- Algorithms can analyze decades of historical data to detect subtle patterns that human traders would likely miss.
- News sentiment analysis by AI provides valuable signals into how markets may react.
Predictive Modeling
- AI algorithms utilize statistical and quantitative techniques to forecast future price movements.
- Machine learning models become more accurate at predictions as they process more data.
- AI can optimize trading timing, manage risk exposure, and predict volatility.
Automatic Execution
- Once AI algorithms generate trading signals, orders can be automatically executed in milliseconds.
- AI enables full automation, from predictive analysis to trade execution, without human intervention.
- Trades can be executed optimally based on variables like liquidity, slippage, and transaction costs.
READ ALSO: Vintage Stock: The Ultimate Entertainment Superstore for Movies, Games, Music and More
Algorithmic Trading Strategies Enhanced by AI
AI empowers algorithms to implement both conventional and more advanced algorithmic trading strategies with greater success.
Trend-Following Strategies
- Identify patterns and follow the momentum of overall market trends.
- AI is adept at detecting macromarket trends, even in noisy data.
Arbitrage Strategies
- Exploit price discrepancies between securities and markets to profit.
- AI can discover even minute pricing anomalies across assets almost instantly to capitalize on arbitrage opportunities faster than any human.
Sentiment Analysis Strategies
- Assess news, social media, and other qualitative data to gauge market sentiment and predict movements.
- Natural language processing by AI parses text data efficiently to determine positive or negative sentiment signals that may impact asset prices.
Algorithmic Hedging Strategies
- Algorithmic systems generate counterbalancing trades to offset risks within a portfolio.
- AI allows more dynamic, precise hedging strategies to mitigate losses.
High Frequency Trading Strategies
- Rapidly execute a high volume of trades to capitalize on small intraday price changes.
- The split-second analysis and trade execution capabilities of AI algorithms make HFT viable and profitable.
Retail Algorithmic Trading Platforms
Initially, algorithmic trading was only conducted by institutional investors and hedge funds. However, AI-powered fintech platforms have now opened algorithmic trading to everyday retail investors.
These platforms provide user-friendly tools that automate elements of the trading process through AI and allow customization based on an individual’s goals and risk tolerance.
Key features retail algorithmic trading platforms offer:
- Robo-advisors – Provide automated portfolio management, asset allocation, and rebalancing through algorithms.
- Automated trading – Enable backtesting trading strategies against historical data before live trading.
- AI analytics – Analyze data, generate insights, and surface trading opportunities.
- Social trading – Allow investors to copy profitable trading strategies from top performers.
- Mobile access – Manage algorithms and oversee trading through mobile apps.
- Account linking – Integrate with brokerage accounts to execute automated trades.
- Customizability – Build personalized trading bots based on defined rules and strategies.
- Paper trading – Simulate trading in real market conditions before risking capital.
Retail platforms like QuantConnect, HedgeTrade, and Numerai are bringing algorithmic trading within the reach of individual investors.
Pros and Cons of Algorithmic Trading With AI
While AI unlocks immense potential, there are also downsides to evaluate:
Pros
- Automates tedious workflows and menial tasks
- Processes data and calculates complex models tirelessly
- Identifies profitable opportunities at superhuman speeds
- Executes trades precisely based on parameters
- Backtest strategies thoroughly across any timeframe
- Tunes strategies continually based on performance
Cons
- Susceptible to overoptimization bias from excessive backtesting
- Subject to algorithmic model risk
- Requires extensive historical data
- Incurs development and infrastructure costs
- Can propagate existing human biases
- Faces regular algorithm updates to avoid going stale
Overall, AI-based algorithmic trading delivers transformative benefits but still requires oversight and expertise to account for its limitations.
The Future of Algorithmic Trading
Looking ahead, here are some potential developments as algorithmic trading continues maturing:
- Automated strategy creation using AI and reinforcement learning without human programming
- Incorporation of unconventional data like satellite imagery and geospatial data
- Mainstream use of deep learning and neural networks to model market complexities
- Wider adoption of AI trading tools beyond hedge funds by retail investors
- More seamless linking between algorithmic trading platforms and brokerages
- Advances in explainable AI models for algorithmic trading
- Stricter regulation around transparency and risks
- Potential disruption of traditional financial institutions
As computing power grows exponentially, the capabilities unlocked by AI will fundamentally reshape markets. Algorithmic trading has already been transformed in just a few decades and will continue to evolve in the years ahead.
To Recap
Algorithmic trading has experienced remarkable growth thanks to artificial intelligence capabilities that unlock greater speed, accuracy, and automation. As computing power progresses, AI promises to propel further advances in predictive modeling, data analytics, and automated execution for algorithmic trading.
Retail algorithmic trading platforms are also democratizing access to sophisticated trading algorithms for regular investors. However, risks remain, and human oversight is still essential. Overall, AI is undoubtedly set to shape the future trajectory of markets dramatically, making algorithmic investing an indispensable element of every trader’s toolkit.
FAQs About Algorithmic Trading and AI
How do you build a trading algorithm?
The key steps are: identifying a strategy, gathering historical data, formulating rules in code, backtesting relentlessly, optimizing based on results, implementing with real market data, executing paper trades, and then deploying the algorithm with real capital.
What percentage of trading is algorithmic?
Estimates put algorithmic trading at over 70% of total equity trading volume in the US currently. Across global financial markets, at least 50-60% of trading is driven algorithmically.
Is algorithmic trading profitable?
Profitability depends greatly on the efficacy of the strategy, the quality of the data, and the efficiency of execution. But algorithms can benefit from backtesting at scale and removing emotional human biases.
What is needed for algorithmic trading?
It requires identifying a strategy, coding skills, historical and live market data feeds, fast infrastructure for strategy computation and order routing, trading platform APIs, and integration with brokerages.
What are the risks of algorithmic trading?
Key risks include overfitting models to historical data, algorithms behaving unexpectedly in live trading, amplified volatility, dependence on quality data, and increased costs for deployment and maintenance.
Does algorithmic trading require machine learning?
Not necessarily. Simple rule-based algorithms can be profitable. But machine learning and AI allow more adaptive, intelligent algorithms that continually improve, reducing overoptimization risks.
In another related article, 5 Best AI Stocks to Buy Now for Long Term Growth
Want to see what stocks the top hedge funds are looking at BEFORE it hits the mainstream news? Get free access for 7 days